5 Proven AI use cases for manufacturers
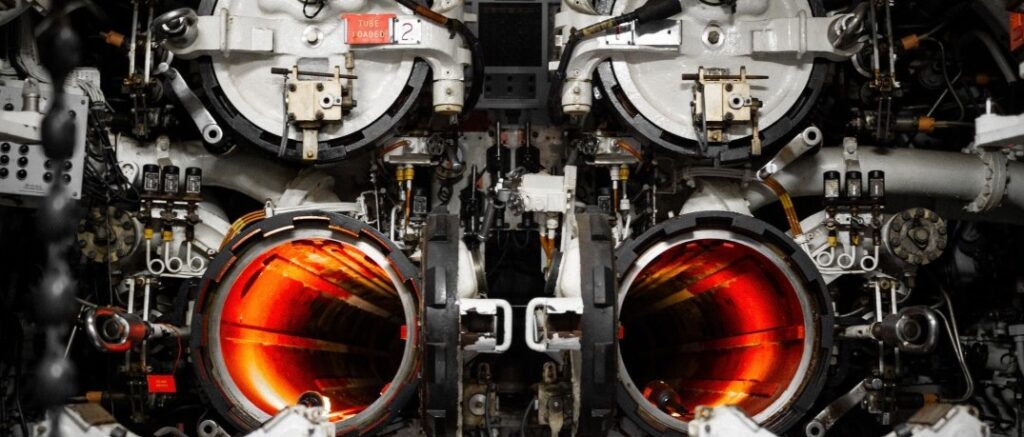
Photo by Philipp Deus on Unsplash
In every sector we see how technology helps some and disturbs other businesses. Market leaders that have been leading for a while get disturbed by new businesses that enter the market which utilize the possibilities of technology better and smarter.
Read in this article what the possibilities with Artificial Intelligence are for your manufacturing business.
Industry 4.0
Modern manufacturing is often referred to as industry 4.0. The Internet of Things (IoT) makes it possible to connect machines and sensors through wired and wireless networks so that we can collect information from the factory floor in real-time.
The application of advanced analytics on this data brings significant advantages to manufacturers. Besides this sensor data, a manufacturer also has other data from different processes throughout the organization. This opens the door to different types of use cases, by applying predictive analytics.
Predictive analytics | Analyzing this data with machine learning and deep learning allows us to forecast problematic situations before they happen and optimize processes for a higher yield. With machine learning and deep learning, we can forecast but also act in real-time where it is needed.
Predictive Maintenance | With predictive maintenance, we aim to forecast the chance that a machine is at risk of failure. There are several ways to measure that a machine is operating differently. Change in operating temperature and a change in the sound a machine is emitting, for instance. With sensors we can monitor this in real-time and use it to form an approximation of the current risk of failure. As a result, we can prevent these failures from happening. For more advanced use cases, where well formed historic data exists, it can also be possible to diagnose what specific part might be at risk of failing.
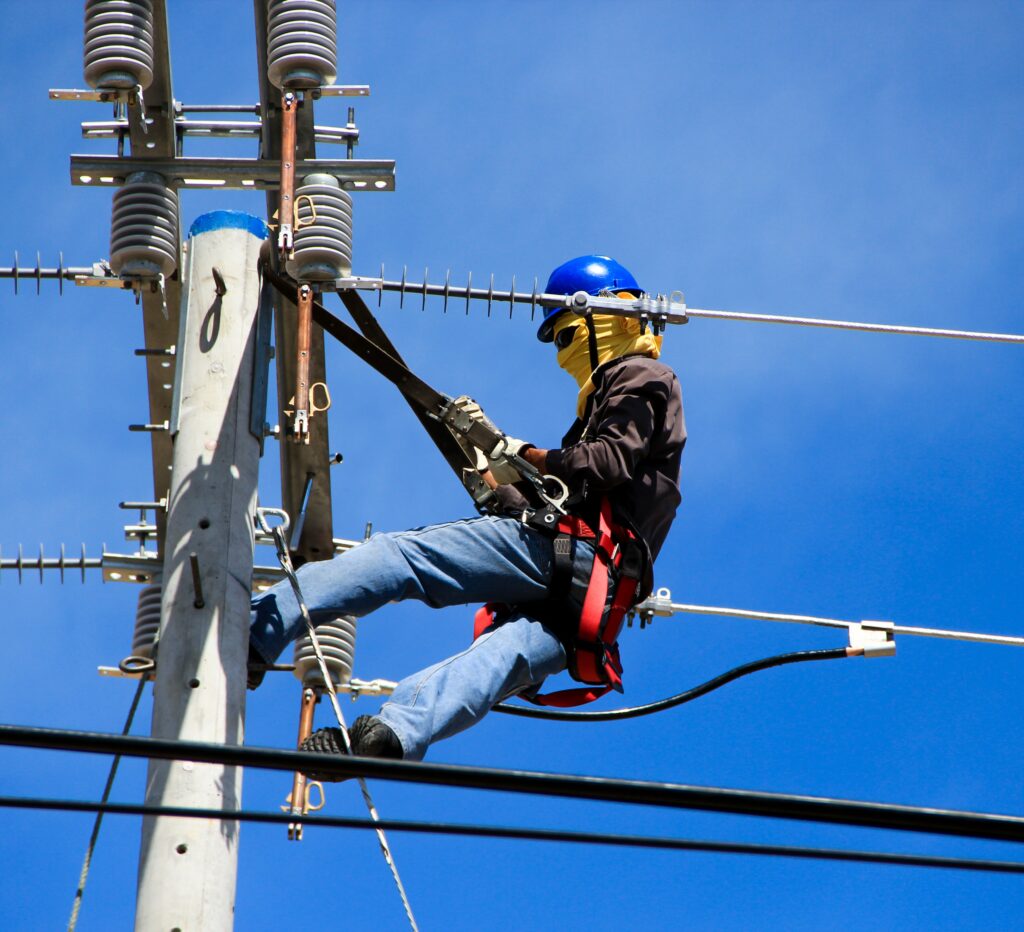
Photo by fran hogan on Unsplash
The model keeps learning from the sensor data and gets better in predicting as we record more events. This often results in an increase of uptime of your machines which allows you to produce more. Beside this it might help you with the planning of your machine maintenance and allows you, for example, to order missing parts in advance to prevent extra downtime. With predictive maintenance, potential issues can be solved before they become a significant problem.
Computer Vision | Automatic quality control can save costs and increase the quality of your product. Computer vision plays a big part in this and can visually check the production for inconsistencies and faults. Quality checks can be performed in real-time, and because it is automated, you can even do quality inspections at multiple points throughout the manufacturing process.
Forecasting Demand & Risk in the supply chain | Forecasting demand helps you control your inventory so that you are never out of stock. You will have a much better understanding of what to have in stock, and what not to have in stock. You can timely contact your suppliers and order what is needed. Predicting delays and localising possible problems in the supply chain allows manufacturers to reduce risks and set up backup plans.
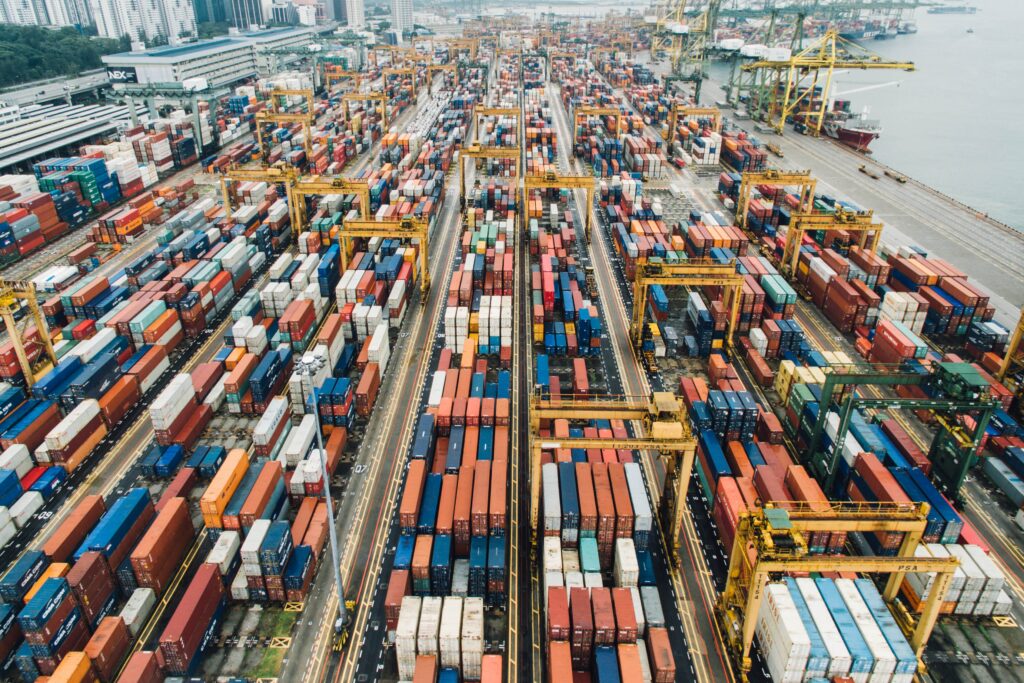
Photo by chuttersnap on Unsplash
There is a lot of benefit to gain with forecasting demand when it is done right. Machine learning makes it possible to combine many different data sources in your forecasts, such as raw material availability, supply chain data, inventory data, account and market data, so that you can produce reliable forecasts.
Price Optimization | With price optimization, we aim to find the best possible price that in return gives the manufacturer an increase in profits. Depending on the available data we use the most suitable analytical method that can predict, for instance, the best time to buy raw materials. Analyzing the costs of production and distribution we reveal the hidden patterns and come up with the most profitable areas to optimize.
Robotic Process Automation (RPA) | RPA is all about automating processes. It is worth noting that RPA is not necessarily involving actual robots; automating processes with software is also considered as RPA. That being said, more and more industrial robots are bought by companies.
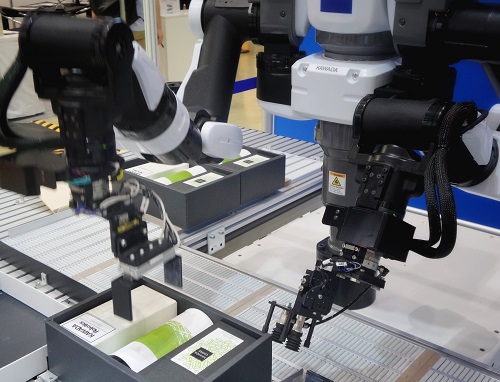
Photo by Franck V. on Unsplash
These robots are used for routine tasks and tasks which might be dangerous for people. The use of these robots empowers the employees and makes more fulfilling work possible while increasing the safety on the floor. Machine learning and deep learning is starting to play a role in both kinds of RPA. For robots, visual recognition and the interpretation of complex sensor data can be crucial in some environments. For process optimization, we might look at using machine learning to automate complex analytical work.
Trifork
At Trifork we work on challenging projects to help our customers take full advantage of a data-driven environment. We deliver complete solutions and take your technology to higher ground. From the start, we make sure that projects are in coherence with the business and the people involved.
More on this topic by Trifork
If you are interested in reading more, please visit the
Trifork webpage on Machine Learning and IoT for Manufacturing
(with a link to our document: “Smart manufacturing with Machine Learning and IoT maximises results by using top technologies”)